Artificial Intelligence
AXA Chairs
United Kingdom
Explainable AI for healthcare: enabling a revolution
Developing technologies that we can trust: a new paradigm for AI
As these limitations have become increasingly apparent, AI experts have expressed the need for a new paradigm, called by some “the third wave”. The three waves of AI refer to consecutive milestones in AI capabilities. According to this division, we are currently experiencing the second wave of AI, dominated by machine and especially deep learning technologies. “It is commonly believed (both inside and outside the deep learning community) that certain progress in AI can only be achieved by combining these statistical learning technologies with other AI technologies, Prof. Lukasiewicz reports. More specifically, the third generation should build on both the first wave AI systems (rule-based or logic-based systems), and the second wave systems. The rationale behind this reasoning is that both AI system waves have complementary strengths and weaknesses when it comes to the different dimensions of intelligence. To put it simply, the first, which is based on handcrafted knowledge, is particularly performant at reasoning, but has no learning capability and poor handling of uncertainty. The “second wave” systems, on the other hand, which are based on statistical learning, “have nuanced classification and prediction capabilities”. They are good at perceiving and learning. “A very natural idea is thus to combine them, the chairholder summarizes, and to create a third-wave of AI systems, that we also call neural-symbolic AI systems”.
Building on the chairholder's expertise in logic-based, neural, and explainable AI as well as on the world-leading medical expertise of the Oxford's Medical Sciences Division, the research team will develop systems that “have an interpretable encoding of logic-based knowledge and a verifiable semantics. They will allow for question answering and analytics in healthcare based on explainable logic-based reasoning, and abstract logic-based domain knowledge will complement the data extraction process, so that the learning process does not require that huge amounts of data anymore. Furthermore, deep learning technologies will be used to allow for easily adaptable and generalizable technologies for extracting structured data from multimodal unstructured sources, and to allow for highly scalable inconsistency- and noise-tolerant reasoning on top of logic-based knowledge”.
The potential for healthcare applications of AI is huge. “It ranges from disease prevention, early detection of diseases, better and more affordable diagnosis, and medical decision making in general to designing new pharmaceutical products and optimized treatments”, Prof. Thomas Lukasiewicz specifies. In this sense, the expected results of this research program will contribute to both the AI and the healthcare community. By moving beyond the limitations of current AI systems, including but not limited to opacity, the output will indeed enable the production of new medical insights and progress. For the insurance industry, these benefits will also allow for a more accurate health risk prediction and the possibility for risk reduction.
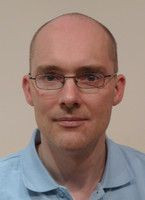
Thomas
LUKASIEWICZ
Institution
University of Oxford
Country
United Kingdom
Nationality
German
Related articles
Artificial Intelligence
Joint Research Initiative
Belgium
Fairness in AI: ending bias and discrimination
Garbage in, garbage out: how to ensure fairness-aware machine learning? When measuring fairness, a natural preliminary question to ask is... Read more
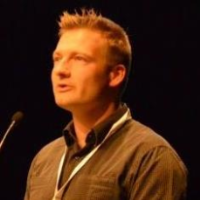
Toon
CALDERS
University of Antwerp
Artificial Intelligence
Joint Research Initiative
Belgium
Fulfilling the potential of AI: towards explainable deep learning
“In its approach of explainable AI, the project will investigate the use of instance-based explanations (explaining the model for one... Read more
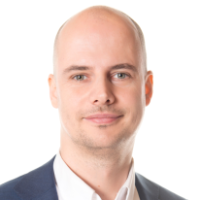
David
MARTENS
University of Antwerp
Artificial Intelligence
Joint Research Initiative
France
Optimizing Pricing in the Insurance Industry : Towards transparent Machine Learning
Opening the black boxes The current research focuses on integrating these advances into a longer and operational value chain to... Read more
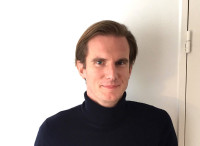
Alexandre
D'ASPREMONT