Modeling & Pricing
AXA Awards
France
An Extreme Value Model For the Analysis of the COVID-19 Pandemic and Its Impact, and the Mitigation of Future Related Crises
The second strand of work will harness the vast wealth of functional data at our disposal to construct estimators for extreme regression risk measures with functional covariates, with a view to analysing and predicting the aftermath of the COVID-19 pandemic. These estimators are so-called “nonparametric estimators”, in the sense that they progressively learn the structure of the data with minimal input from the user. The methods will be implemented on simulated and real data from climatology, epidemiology, insurance, and finance. This requires specialised technical skills and cutting-edge results from extreme value theory.
With this combination of novel risk measurement techniques and high-dimensional data methods, this research program will provide a diverse toolbox for the assessment and mitigation of extreme risk due to rare events, including a pandemic or a disruptive climate event.
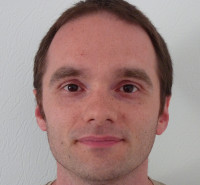
Gilles
STUPFLER
Institution
École Nationale de la Statistique et de l'Analyse de l'Information
Country
France
Nationality
French
Related articles
Modeling & Pricing
Joint Research Initiative
Canada
Use and Value of Unusual Data in Actuarial Science
A Joint Research Initiative between Université de Québec à Montréal and AXA Group Actuarial Function Satellite Images The weak correlation... Read more
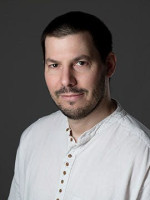
Arthur
CHARPENTIER
Université du Québec à Montréal
Modeling & Pricing
Joint Research Initiative
France
Towards simpler numerical methods for the management of assets and liabilities
Reducing calculation time, without extrapolating To do so, the project aims to develop new dedicated algorithms for the ALM, called... Read more
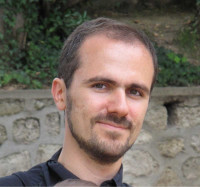
Aurélien
ALFONSI