Artificial Intelligence
Joint Research Initiative
France
Optimizing Pricing in the Insurance Industry : Towards transparent Machine Learning
Opening the black boxes
The current research focuses on integrating these advances into a longer and operational value chain to exploit them for actual pricing and overall profit and loss. “What we did in the first JRI is going to be a block of what we do in the second one. The algorithms we’ve developed so far are intrinsically local in the sense that they can only model the probability of one prospective client to sign the contract. Optimizing prices solely based on individual decisions is of little interest for an insurer. Our objective now is to understand how to optimize all price proposed to a pool of prospects, while managing other key aspects of contracts and enforcing advanced constraints on overall sales, risk, profit, and interpretability”.
The added value of this partnership will be to test the theory on real-life data. “At AXA, we hold a lot of client information, so we have the resource for accurate predictions. It’s really gratifying for an actuary like myself to have the opportunity to contribute to the actuarial theory ”, comments Guillaume Beraud-Sudreau. For Prof. d’Aspremont too, the bilateral approach provides something of great value: “witnessing how deep theory research is translated into applications is always very gratifying.”
Today it is pretty standard to make predictions with machine learning. But making the predictions explainable is highly innovative. Transparency inherently offers more reliability, flexibility and thus, more actionability. In this sense, the accumulated output of both the JRIs mentioned above will contribute to unlocking the potential of machine learning in the insurance sector, as well as for the policy holders, with the promise of more accurate and fairer premiums.
* Kamet Ventures is a €100m InsurTech incubator dedicated to conceptualizing, launching and accompanying disruptive products and services for insurance clients.
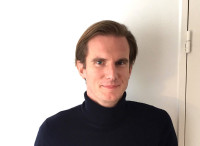
Alexandre
D'ASPREMONT
Institution
Ecole Normale Supérieure
Country
France
Nationality
French
Related articles
Artificial Intelligence
AXA Chair
United Kingdom
Explainable AI for healthcare: enabling a revolution
Developing technologies that we can trust: a new paradigm for AI As these limitations have become increasingly apparent, AI experts... Read more
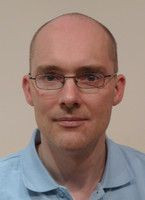
Thomas
LUKASIEWICZ
University of Oxford
Artificial Intelligence
Joint Research Initiative
Belgium
Fairness in AI: ending bias and discrimination
Garbage in, garbage out: how to ensure fairness-aware machine learning? When measuring fairness, a natural preliminary question to ask is... Read more
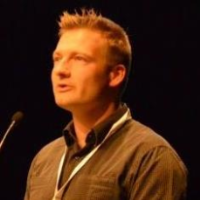
Toon
CALDERS
University of Antwerp
Artificial Intelligence
Joint Research Initiative
Belgium
Fulfilling the potential of AI: towards explainable deep learning
“In its approach of explainable AI, the project will investigate the use of instance-based explanations (explaining the model for one... Read more
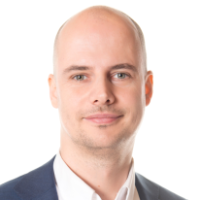
David
MARTENS