Artificial Intelligence
Joint Research Initiative
Belgium
Fulfilling the potential of AI: towards explainable deep learning
“In its approach of explainable AI, the project will investigate the use of instance-based explanations (explaining the model for one prediction)”, specifies prof. Martens. These will be obtained using the counter-factual concept: what data, if it were not present, would have led to a different decision? “Instance-based explanations provide a data-driven explanation for a single prediction." Martens explains, "For example: what words/paragraphs said in a phone call to a help desk made the AI predict that the customer is not satisfied with his or her service?” So, for this example, the approach would consist in identifying which words, if they would not have been said in the call, would have led to a satisfied (or neutral) prediction. He further will look at how such explanations can be used to obtain general insights into the domain.
“To achieve ethical data science, transparency is at the basis of everything. Fairness and accountability depend on it. If you want to make sure your AI system doesn’t discriminate, it is crucial to be able to explain. I’m very excited to work on this issue with AXA. Not only does this collaboration provide us with large amounts of real-world data, it also ensures that the research and technical work we do will have a concrete impact.” Insists prof. Martens. Indeed, once the ExAI algorithms are developed, they will be validated through use cases within AXA, both in the house insurance sector: the first would be a model to predict the likely cost of household damage; the second, as in the previous example, to detect the satisfaction level of customers, based on calls, mail/letter data, complaint forms, etc.
AI is emerging as the defining technology of our age, opening social and economic perspectives we never thought possible. However, for the benefits to be truly and safely accessible, key challenges remain, one of which is transparency. The present JRI offers the rare opportunity of combining research and practitioner expertise in order to make both academic and practical advances in that area. Focusing on insurance business applications, the output of the project will greatly contribute to the safe and responsible usage of the most advanced AI technologies for real-world applications in the future.
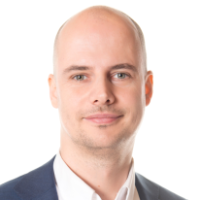
David
MARTENS
Institution
University of Antwerp
Country
Belgium
Nationality
Related articles
Artificial Intelligence
AXA Chair
United Kingdom
Explainable AI for healthcare: enabling a revolution
Developing technologies that we can trust: a new paradigm for AI As these limitations have become increasingly apparent, AI experts... Read more
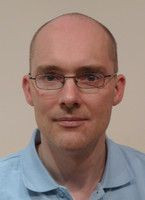
Thomas
LUKASIEWICZ
University of Oxford
Artificial Intelligence
Joint Research Initiative
Belgium
Fairness in AI: ending bias and discrimination
Garbage in, garbage out: how to ensure fairness-aware machine learning? When measuring fairness, a natural preliminary question to ask is... Read more
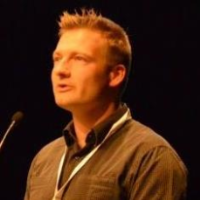
Toon
CALDERS
University of Antwerp
Artificial Intelligence
Joint Research Initiative
France
Optimizing Pricing in the Insurance Industry : Towards transparent Machine Learning
Opening the black boxes The current research focuses on integrating these advances into a longer and operational value chain to... Read more
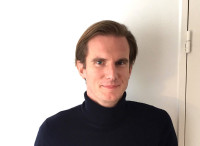
Alexandre
D'ASPREMONT