Artificial Intelligence
Joint Research Initiative
Belgium
Fairness in AI: ending bias and discrimination
Garbage in, garbage out: how to ensure fairness-aware machine learning?
When measuring fairness, a natural preliminary question to ask is how to define it. Building upon previous research (Friedler et al. 2016), prof. Calders works on the assumption that giving an exact and generic definition of fairness is an impossible task, and that consequently, measures of fairness should be situation dependent. “We consider that constructing a definition of what is fairness in AXA’s operational context is part of the project itself, he explains. Once this specific definition is constructed, the project will pursue its second objective: find methods to assess the level of fairness of AXA’s existing decision procedures. “One simple approach could be to assume that men and women should have equal access to low insurance premiums. Then we would just have to compare percentages. However, this approach does not work in this case, because a correlation between gender and accidents has been proven. In other words, it wouldn’t be fair.” The approach that will be adopted is slightly different. “A better way is to look at people with a similar level of premium, say high, and see how many of them were involved in actual accidents. If your prediction is correct, you would expect to see high probability in this group. Now, if you split these high premiums into genders, and see that the number of accidents is much higher for men than women, you will be able to tell that the system is biased”. For ethnicity, the problem becomes yet more complicated. Indeed, gender is a characteristic that is stored in insurance data sets, ethnicity, on the other hand, isn’t. “That’s a crucial challenge we want to deal with in this project. How do we assess whether an insurance discriminates on ethnicity, if we, ourselves, can’t make out the difference in the input data? For all we know, the algorithms could be discriminating on names, schools, neighbourhoods, but that is much harder to examine. Our solution is to create some kind of synthetic population, with artificial profiles, and then to run the algorithms as if they were real”.
Using this approach, the project ultimately aims is to obtain a set of compatible and effective measures that reflect the type of fairness that AXA wants, while at the same time, obeying increasingly severe data protection laws. In particular, the European Union has one of the strongest anti-discrimination legislations. In fact, the recent General Data Protection Regulation (GDPR) explicitly mentions profiling as an activity in which decisions should not be based on personal data and suitable measures should be in place to safeguard the data subject’s rights and freedoms and legitimate interests.
The present JRI effort was precisely initiated in anticipation of the enforcement of such regulations. In the near future, companies will be increasingly asked to answer for their decisions made by algorithmic systems. In this context, it will primordial to have mechanisms in place to continually screen decision prediction and make sure they are not biased. “Historically, research on fairness and machine-learning has stayed very academic. Here, the JRI offers the opportunity to confront the issue with real-life scenarios and cases. This is a big motivational boost for me, says prof. Calders. Not only does it help the research align with reality, but it also makes sure it will have an impact”.
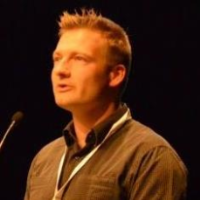
Toon
CALDERS
Institution
University of Antwerp
Country
Belgium
Nationality
Belgian
Related articles
Artificial Intelligence
AXA Chair
United Kingdom
Explainable AI for healthcare: enabling a revolution
Developing technologies that we can trust: a new paradigm for AI As these limitations have become increasingly apparent, AI experts... Read more
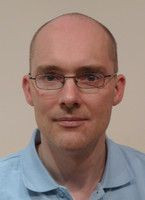
Thomas
LUKASIEWICZ
University of Oxford
Artificial Intelligence
Joint Research Initiative
Belgium
Fulfilling the potential of AI: towards explainable deep learning
“In its approach of explainable AI, the project will investigate the use of instance-based explanations (explaining the model for one... Read more
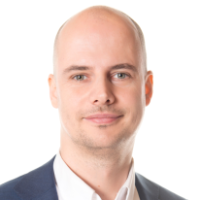
David
MARTENS
University of Antwerp
Artificial Intelligence
Joint Research Initiative
France
Optimizing Pricing in the Insurance Industry : Towards transparent Machine Learning
Opening the black boxes The current research focuses on integrating these advances into a longer and operational value chain to... Read more
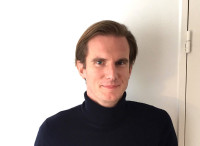
Alexandre
D'ASPREMONT