Natural Catastrophes
Post-Doctoral Fellowships
France
Preventing avalanches and snow-melt related floods by improving forecasting systems
To prevent disaster from happening, different state-of-the-art forecasting systems have been developed. These systems rely on detailed simulations of snowpack physical properties and their evolution in regard to factors such as meteorological conditions, local topography or ground characteristics. However, current snowpack simulations are not 100% reliable. Significant discrepancies between real and simulated snowpack occasionally alter the systems' capacity to anticipate potentially devastating events. "Uncertainties in snowpack simulations accumulate over the snow season, since the snowpack has an important memory of past meteorological events. Nowadays snowpack simulations are not corrected with snow observations; mainly due to lack of daily distributed information on the snowpack. But new possibilities in regard to real-time monitoring of snowpack distribution are showing good results and could be exploited for rectifying deviations in snowpack simulations", explains Dr. Jesús Revuelto. Building on the latest advances in snow science, the researcher's project aims to refine the spatial resolution of snowpack simulations and reduce simulation deviations by enabling the obtention of both large-scale and refined real-time data on snowpack distribution. The ultimate objective is to further improve snowpack forecasting capabilities by enhancing the reliability of forecast systems in mountain areas.
"Accurately representing snowpack evolution over large areas is a challenging task because each method and technology has its limitations", explains Dr. Jesús Revuelto."Field measurements are the most accurate among snowpack observations, but their poor spatial extent makes them inappropriate for large-scale data assimilation", the researcher describes."On the other hand, remote sensing data, including satellite data, enable the obtention of information over large areas, but this time, the spatial resolution is limited." Indeed, optical satellite sensors that retrieve daily data from snow-covered areas have a spatial resolution of 250 to 500 m and are affected by cloud presence. "The satellite information obtained at these spatial scales cannot represent the high spatial variability of the snowpack distribution in smaller distances, particularly for pixels in the satellite image which are partially snow cover", Dr. Jesús Revuelto specifies. "Downscaling snowpack simulations to a spatial resolution that accurately represents the small scale spatial variability of the snow is crucial for improving avalanche and flood hazard forecasting because terrain features which control local snow accumulation can have major importance on many endangering situations."
Using field investigations to better interpret satellite images
To overcome these obstacles, the project intends to use field analysis of small scale topographic control on snow distribution to better understand and interpret satellite images. Dr. Jesús Revuelto and the team in which he will be hosted, will focus their field investigations in the Arve Valley located in the western European Alps. Studying the topographic drivers of snowpack distribution in the area, they intend to combine the newly-gained insight with careful study of satellite data to determine snowpack variability within each pixel "Several factors need to be taken into account: the incidence angle of the sun, terrain slope, the presence of vegetation, etc", explains Dr. Jesús Revuelto. "Using insight into the topography of mountain areas, the sensitivity on determining the snow cover distribution obtained from the satellite sensors can be increased by a factor of ten, from 250 m to 25 m", he reports. Assimilating this method into the state-of-the art Crocus snowpack model – a numerical model which has been run operationally for simulating snow dynamics and forecasting avalanches over the French mountains for 30 years –, deviations in the prediction of floods and snow avalanches can be considerably reduced.
Mountains are among the most sensitive areas to climate change. Improving snowpack modelling is crucial to a better mitigation capacity of snow-related hazards such as snow-avalanches and floods, which will likely become more frequent with climate change. By aiming to refine the spatial resolution of snowpack simulation and to improve simulation performance assimilating optical satellite data, Dr. Jesús Revuelto's project promises substantial advance in snow science. Furthermore, the envisaged collaboration with Météo France will provide a great opportunity to rapidly feed his findings into operational forecasting.
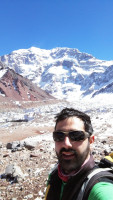
Jesus
REVUELTO
Institution
Centre National de la Recherche Scientifique
Country
France
Nationality
Spanish
Related articles
Natural Catastrophes
Joint Research Initiative
Spain
Understanding Wildfire Risk: A Local-Scale Assessment Framework in Chile
Wildfire is a major hazard across the globe, causing extensive economic, ecological and health impacts. While wildfires are common throughout... Read more
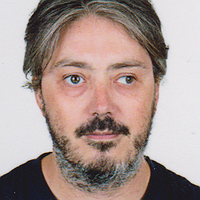
Marcos
RODRIGUES MIMBRERO
University of Zaragoza
Natural Catastrophes
Post-Doctoral Fellowship
Italy
Strengthening Coastal Communities’ Resilience Through Better Forecasting and Projecting Compound Flood Risk
Between March 4 and 15, 2019, tropical cyclone Idai hit Mozambique twice. This storm, of rare intensity, was one of... Read more
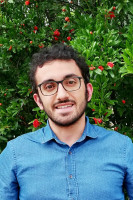
Andrea
FICCHì